The rise of new AI-powered tools is changing the way organizations operate. And for enterprises, agentic AI can transform your business at a fundamental level.
JPMorgan Chase can attest to the power of agentic AI—it’s already saving the organization 360,000 hours of manual review a year. As can AT&T, which reduced operating expenses by 15%. Meanwhile, Amazon and Netflix used agentic AI to increase customer retention by 20% and 10%, respectively.
If your enterprise isn’t preparing to join these AI leaders, you risk falling behind the competition.
What is agentic AI?
Agentic AI refers to artificial intelligence systems that have goal-directed behavior and can take autonomous actions to achieve those goals. In other words, agentic AI solutions are designed to take initiative and set and pursue goals, while continuously learning and adapting based on new information.
Agentic AI systems are much like human employees, rather than traditional software or AI systems, in that instead of focusing on singular tasks, agentic AI programs can handle many responsibilities and perform diverse functions.
Organizations that adopt agentic AI can use this technology to do more than just respond to narrowly defined requests. Agentic AI can identify what an employee needs to do based on natural language queries—and determine what it needs to do to help the employee achieve their goals. This is especially useful for tasks that require adaptability and multi-step problem solving, like creating user accounts, changing passwords, and getting purchase order details.
In other words, an agentic solution moves beyond reactive interactions and becomes a partner (and AI copilot) that drives meaningful results.
Understanding agentic AI should be at the top of your list if you’re responsible for selecting, implementing, or guiding new investments. Agentic AI isn’t just a new technology you should get around to learning when you have the chance—it’s a new way of thinking about how AI can solve problems, improve operations, and drive better results across your entire organization.
Traditional AI vs. agentic AI
Traditional AI—often described as narrow or basic—usually addresses specific problems in a narrow scope. For example, traditional AI may categorize support tickets, analyze text sentiment, or respond to a routine query. It’s very effective at handling singular use cases, but that’s where its capabilities typically end.
Agentic AI, on the other hand, goes beyond single-function tasks, supporting a much wider variety of complex, diverse workflows. These systems can connect to multiple apps, databases, and internal systems to coordinate tasks that span multiple departments.
For example, a new user in an organization may not have access to an app required to do their job. This user can submit a ticket, and the AI agent can determine the appropriate permissions and give them access to the required application.
Agentic AI vs. generative AI: What’s the difference?
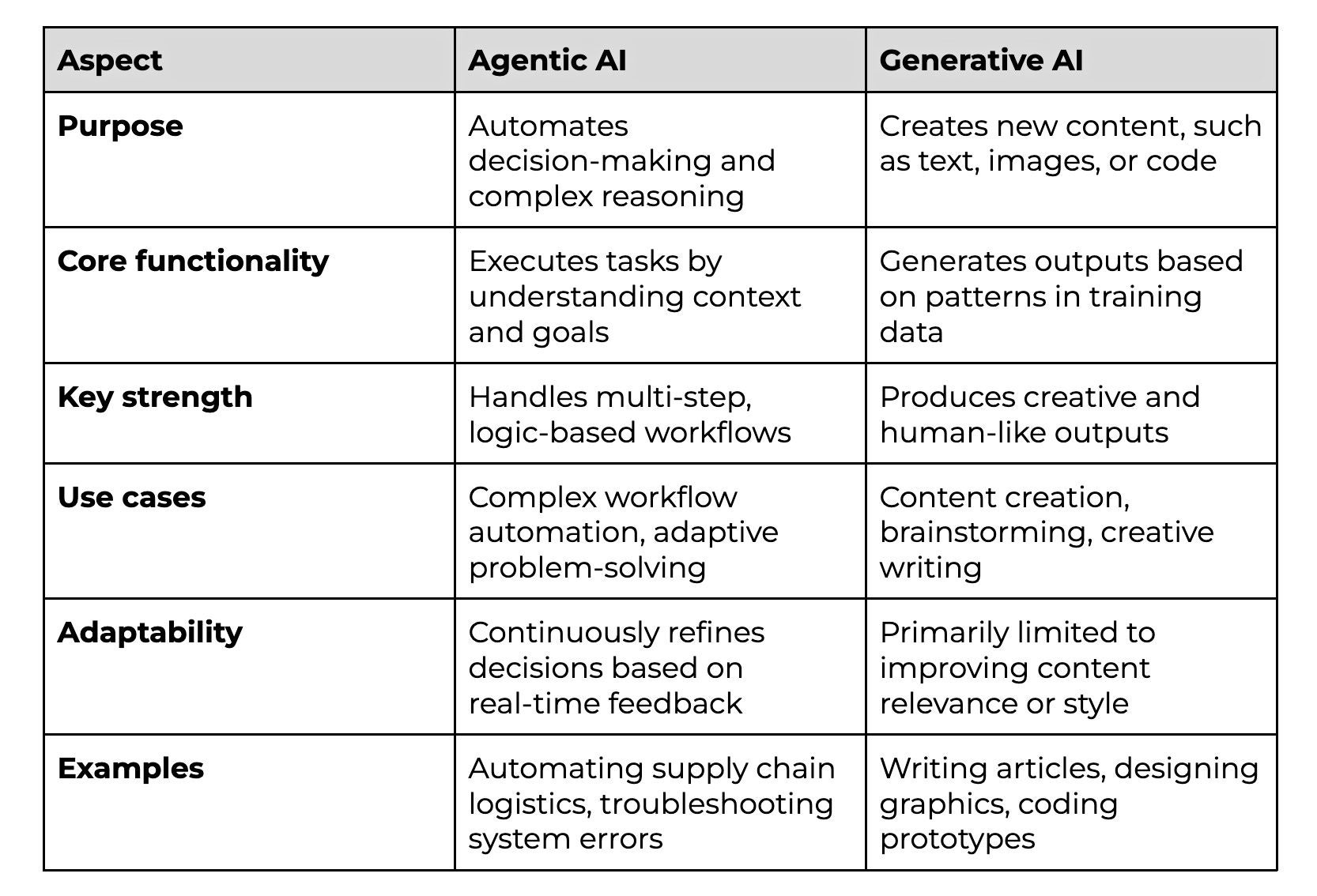
Generative AI is an AI model that allows the end user to generate content based on its training data, which includes text, images, or other content. The type of content generated depends on the model and the specific data it’s trained on.
Generative AI responds to user prompts using natural language processing (NLP) to understand what the user wants and produce the expected output. This is useful for tasks like generating marketing copy, brainstorming product ideas, and writing code.
Agentic AI takes generative AI a step further, using generative AI models to understand what the user wants and take that output to pursue goals. Agentic AI does this by:
- Identifying what the user needs in their workflow
- Tapping into external datasets to gather information
- Proposing a solution that solves the user’s request
- Taking action to complete the user’s goal
Instead of only suggesting a solution to the user, AI agents can take strategic action and work with multiple tools to complete tasks autonomously.
What is an AI agent?
An AI agent is an individual entity designed to perceive its environment, reason based on input from the user, and act autonomously to achieve a desired result. AI agents can be as simple as an AI assistant connected to internal systems or as complex as self-driving workflows that operate autonomously.
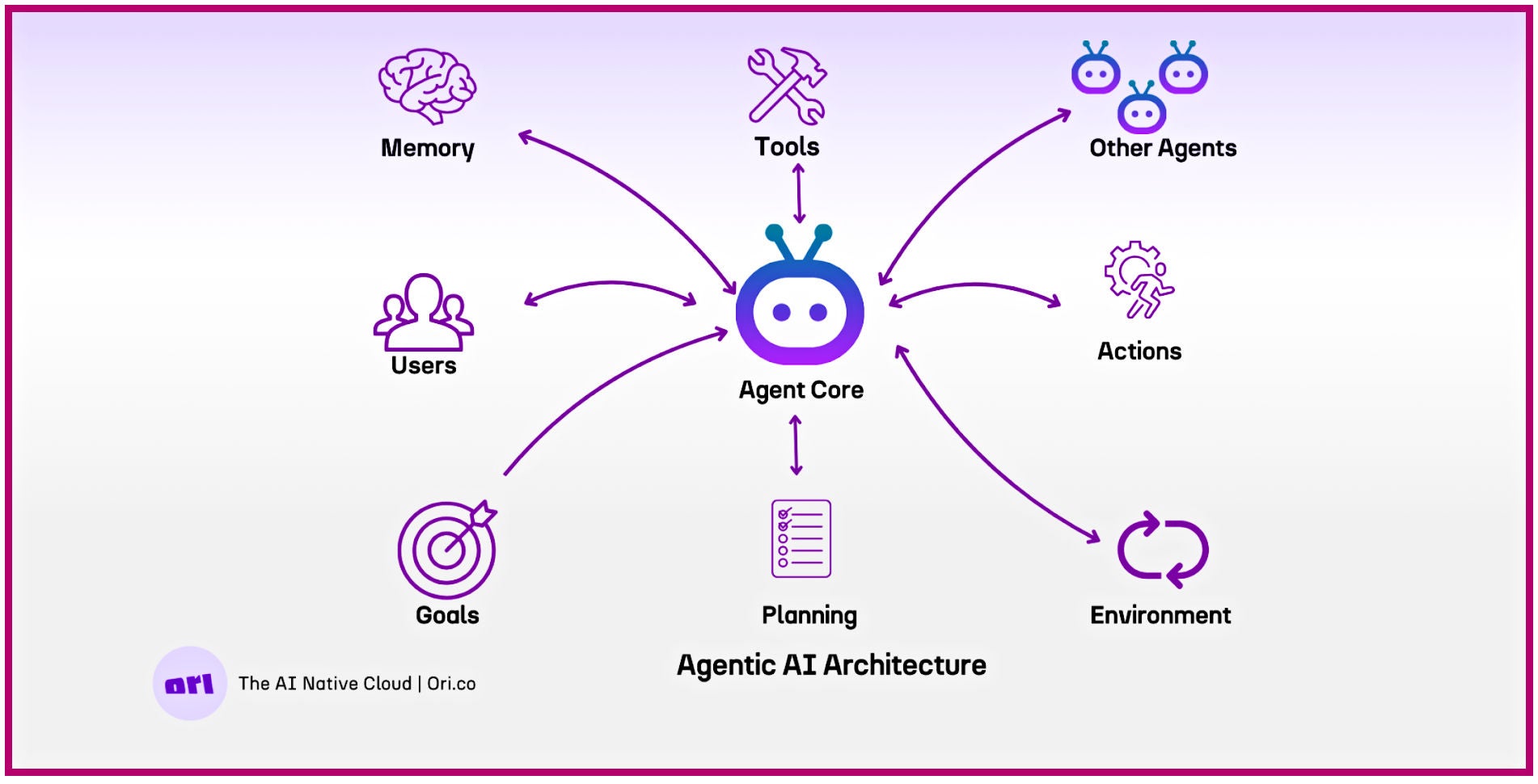
Agentic AI may use multiple types of AI agents that work together to achieve more complex tasks. For example, you can expand on a simple HR chatbot's capabilities by creating additional agents to handle onboarding, employee payroll questions, HR information retrieval, and employee retention suggestions. This AI will observe its environment and adapt as your business changes to better achieve its goals—all without human intervention.
How agentic AI works
The core of agentic AI is its ability to take autonomous actions based on the goals it is programmed to achieve. An agentic AI system doesn't just react to commands; it can plan, predict, and adjust its behavior over time. These AI systems can process large amounts of data, identifying patterns and relationships that guide their decisions without constant human input.
Agentic AI relies on a combination of several technologies, including machine learning (ML), large language models (LLMs), and NLP. Machine learning and LLMs help the system understand complex data, generate insights, and act accordingly. NLP is there to help the system understand user input and process it using the AI model.
But those technologies can only go so far without additional input. AI agents work best when you supplement the data an AI model is trained on with current information using retrieval augmented generation (RAG). RAG is a technique that enhances AI models' capabilities by combining their reasoning and problem-solving abilities with real-time data retrieval. This enables agentic AI to handle things like:
- Automated customer support: It can adapt based on the customer’s specific problem, using RAG to pull the latest product updates or FAQs for more accurate support.
- Supply chain optimization: By combining ML and real-time data retrieval, AI can monitor inventory levels, predict demand surges, and reroute shipments during delays.
- Dynamic workforce management: Agentic AI can plan and adjust staff schedules based on real-time data like employee availability, workload forecasts, and unexpected absences.
- Fraud detection: Agentic AI systems can autonomously flag suspicious transactions using pattern detection, adapting their algorithms in real time as fraud techniques evolve.
- Proactive ITSM: IT teams use agentic AI to monitor network performance, identify potential failures, and implement fixes before issues escalate, using RAG to pull the latest security patches and diagnostics.
Agentic AI can work without RAG, but it's limited by the base capabilities of the language model and may not have the most up-to-date data. RAG allows AI agents to produce more informed, on-demand results.
AI history: The rise of agentic AI
AI has evolved from rule-based systems into today’s advanced models that learn over time and adapt to constantly changing conditions. Rule-based systems work amazingly well with narrow, well-defined tasks, such as answering a rigidly defined question, but the rule-based approach limits how much organizations can rely on it for more complex workflows.
The rise of generative AI changed that. Unlike rule-based systems, generative AI leverages LLMs to process and interpret user input in natural language, allowing it to generate creative, contextual outputs and determine relevant actions.
Generative AI’s initial capabilities revolved around content creation, such as drafting marketing copy, automating customer responses, and generating images or code. But when it’s combined with agentic AI systems that can take direct, autonomous action, it forms a dynamic, adaptable system that can understand and interact with sophisticated workflows. For example, an AI agent can now generate a personalized customer email, retrieve relevant order details, and initiate a refund request—all without human intervention.
That’s why 65% of companies in a recent survey are adopting generative AI and AI agents. With these advancements, AI is no longer limited to handling basic, repetitive tasks. It’s now redefining how organizations operate and enabling more adaptive, intelligent, and scalable workflows.
Learn more about how agentic AI is leading to the next evolution of enterprise AI.
The 5 benefits of agentic AI
Agentic AI isn’t just a buzzword—it’s a fundamentally different approach to AI that will help enterprises stay ahead of the curve. Since AI agents can proactively learn, adapt, and solve problems, your organization will be able to operate in a more efficient and intelligent way. Here are a few examples of the benefits you’ll see when using agentic AI in your organization.
1. Efficiency and productivity improvements
The number of tasks enterprises constantly handle increases as the company grows. Complex workflows can slow down even the most productive members of your team, especially when they have to do the same tasks over and over again and manually dig through information to learn.
Agentic AI can enhance your company’s efficiency and increase your team’s productivity by automating workflows throughout the organization and delivering on-demand, self-service support. AI can process large amounts of data, meaning many tasks are now accomplished in seconds. By autonomously managing tedious and time-consuming manual tasks, agentic AI enables your teams to focus more on strategic, revenue-driving initiatives.
2. Enhanced support operations
It’s not uncommon for support teams to constantly juggle repetitive tasks like password resets, information lookups, account creation, and data analysis. AI agents can manage many of these tasks, reducing the overall burden of your support team.
As a result, your support team can get much more done in less time and make fewer errors. Instead of spending hours every week handling common issues, employees can focus on more impactful work and leave redundant tasks to their AI systems.
Automating key workflows can also improve job satisfaction and morale. Employees won’t get bored doing the same tasks over and over again. Instead, they can learn and work on higher-level projects, helping them improve their skills and advance in your organization.
3. Greater flexibility and scalability
One of the biggest challenges with traditional AI is its limited scope. Narrow AI software is limited to specific tasks and follows a rules-based approach. If you want it to handle other business functions, you have to add in more specialized tools. This is still useful but faces challenges in more complex workflows.
AI agents offer organizations far greater flexibility. For starters, since agentic AI can handle more complex tasks, it can help scale support operations during busier times (such as the holiday season for retail). Organizations won't need to hire as many additional temporary staff and can use process automation to allocate resources during those times to handle the increased workload.
The flexibility of AI agents also offers a wider range of use cases. Since many AI agents use RAG, they can continuously take in new information to ensure the AI’s output and direction are correct. Updated information allows AI agents to adjust workflows as changes occur, instead of requiring software updates and new AI software.
4. Data-driven decision making
A key advantage of AI is its ability to analyze large amounts of data. Agentic AI can gather, analyze, and learn from data—helping your enterprise predict trends, discover challenges, and take advantage of new opportunities. It does this by gathering data from your chosen sources, processing it, and training AI models that understand your data.
It isn’t just a matter of generating reports based on predefined output, either. Users can ask AI agents questions and quickly learn what they need to make data-informed decisions. You can also integrate these insights into your existing AI agent workflows, helping them make better decisions without requiring human input.
5. Faster development cycles
Agentic AI is also useful for developers and engineers who work on complicated problems for your organization. For example, developers can use AI agents to assist with programming. AI can analyze code repositories to learn about your software, allowing developers to learn about your code base, troubleshoot software bugs, and develop new program features.
Taking out a lot of the programming work allows your developers to speed up the development process, optimizing what already exists and quickly shipping new features. It enables your team to adapt to the changing market and gain greater agility, giving you a competitive advantage.
Agentic AI in action: Agentic AI use cases and real-world examples
Agentic AI isn’t limited to a single department. Its ability to operate autonomously, set goals, and perform tasks makes it invaluable across your entire enterprise. From HR to engineering, agentic AI provides a holistic solution that helps your organization achieve its strategic objectives.
1. Human resources
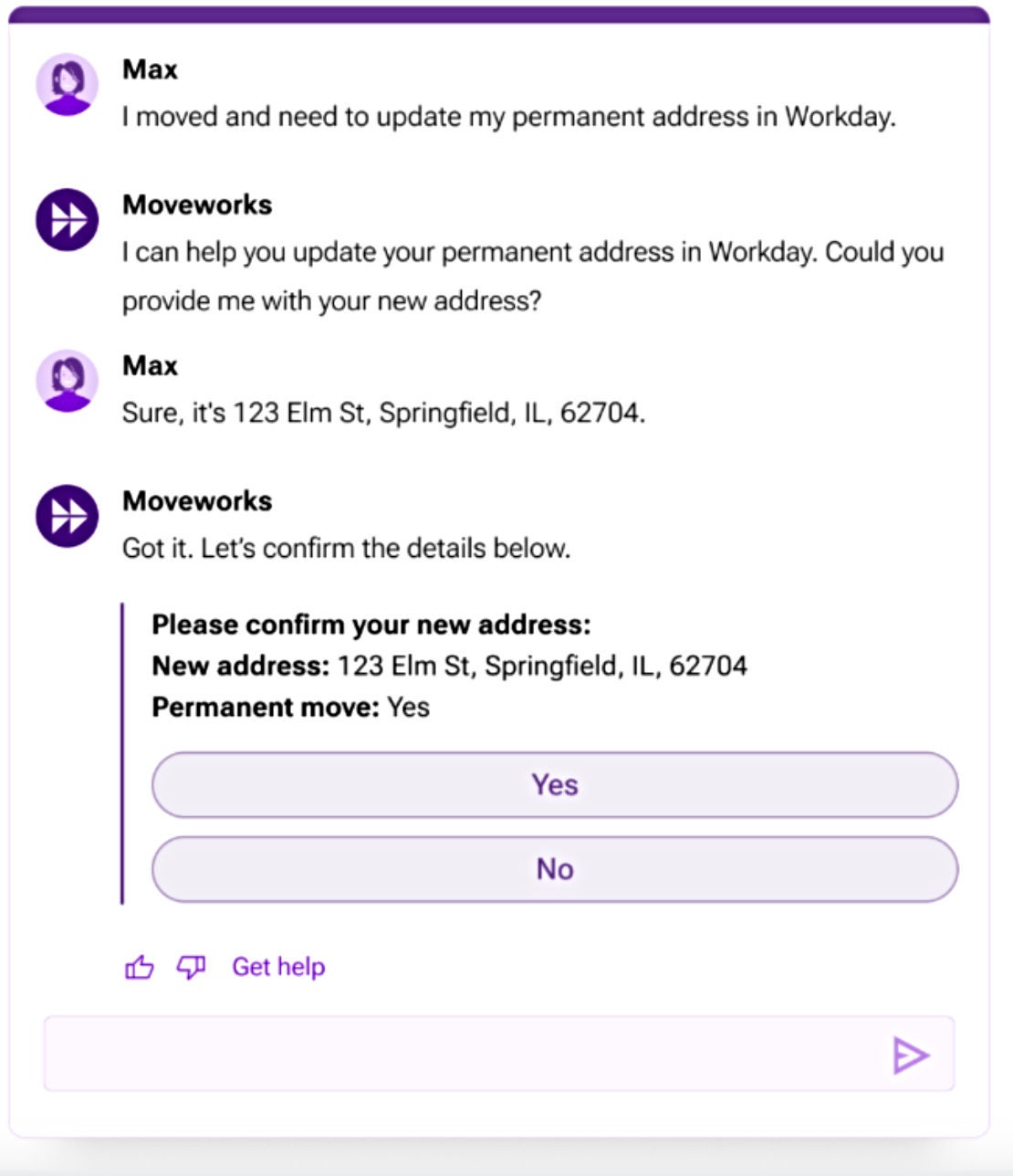
Agentic AI is useful for HR operations because it can streamline common tasks. For example, you may have a standard procedure for onboarding employees, including setting up user accounts, emailing materials, and checking in with employees to make sure they have everything they need. You can build AI agents that automate these tasks and others—like approving time off, responding to support requests, and managing payroll.
This frees up your HR team to focus on more strategic tasks. Instead of manually dealing with routine questions and onboarding tasks, your team can assess current procedures and data to develop strategies to improve employee retention and better manage compliance.
2. ITSM
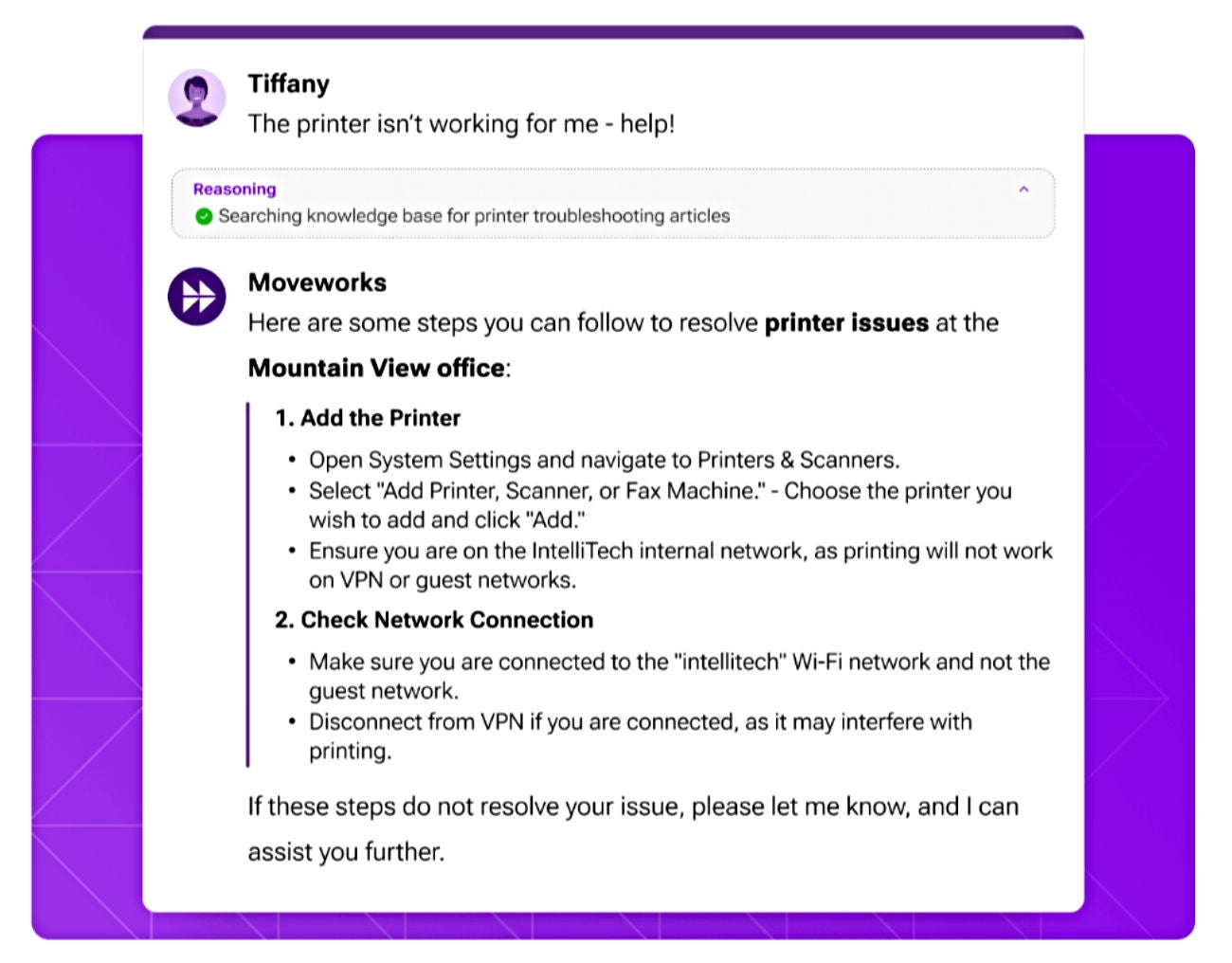
Your IT service management team likely spends their days handling routine issues, such as password resets, PC troubleshooting, pushing software updates, and other IT tasks. AI agents can handle many of these tasks on their own, only looping in human IT support for more complex issues.
Traditional AI may be able to assist with some IT tasks, such as simple questions through chatbots or ticket classifications for team members. But when you add agentic AI for ITSM to the mix, you get a tool that can go beyond simple classification and summarization—something that can actively figure out the user’s request or problem and take action to resolve the ticket or maintain your infrastructure.
3. Finance
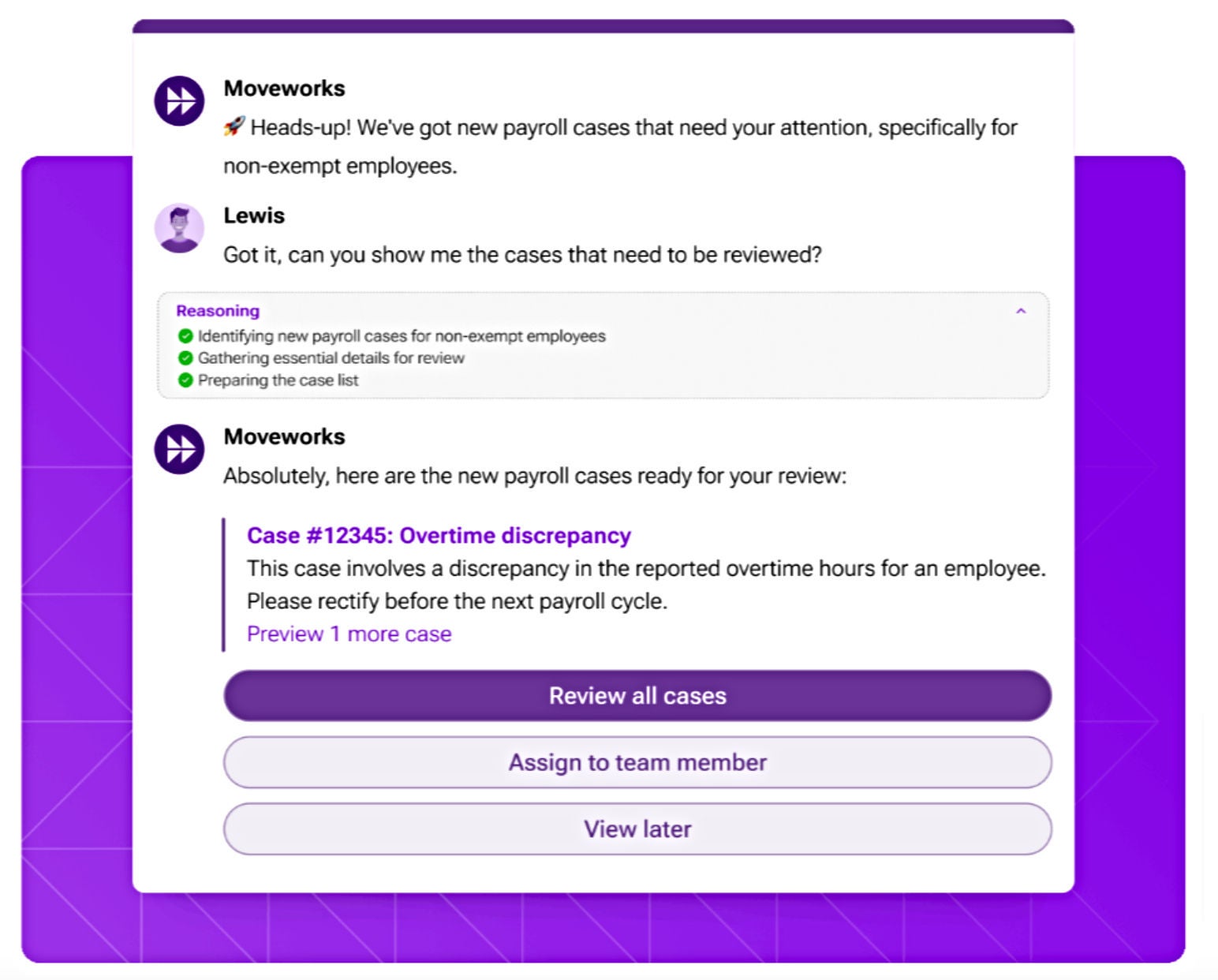
Agentic AI solutions for finance offer many benefits for internal departments and institutions. Some traditional solutions can do basic tasks like summarizing data or automating data entry and retrieval, but they fail to handle more complex tasks that require multiple steps.
In comparison, AI agents can manage tasks like payroll processing, complex data analysis, and processing expense reports. On top of that, AI agents can analyze large amounts of data to look for anomalies that may indicate problems (such as fraud or data entry errors).
Streamlining these operations helps organizations identify and resolve potential issues before they impact the business and gain more insights to improve their overall financial performance.
4. Sales
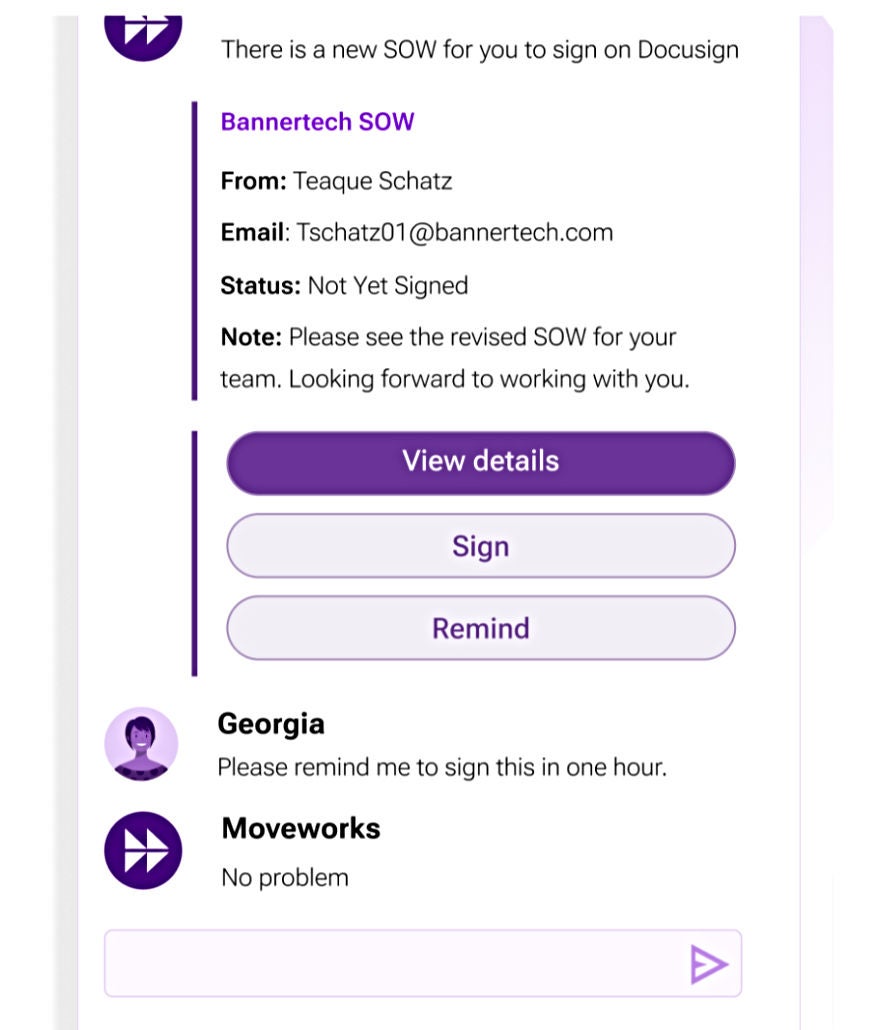
Adding AI agents to sales tasks is a great way to improve your team’s productivity and help them service their clients. You can use traditional AI to help gather information about customers and process basic information. But it struggles to help with more complicated workflows, such as examining your sales pipeline and generating more advanced insights from data in your customer relationship management (CRM) system.
Agentic AI, on the other hand, can gather details from your databases and other sources to maintain your CRM data and offer insights that your sales team can use to close more deals. It can also help respond to emails, answer basic questions, and take corrective actions without human intervention.
5. Engineering
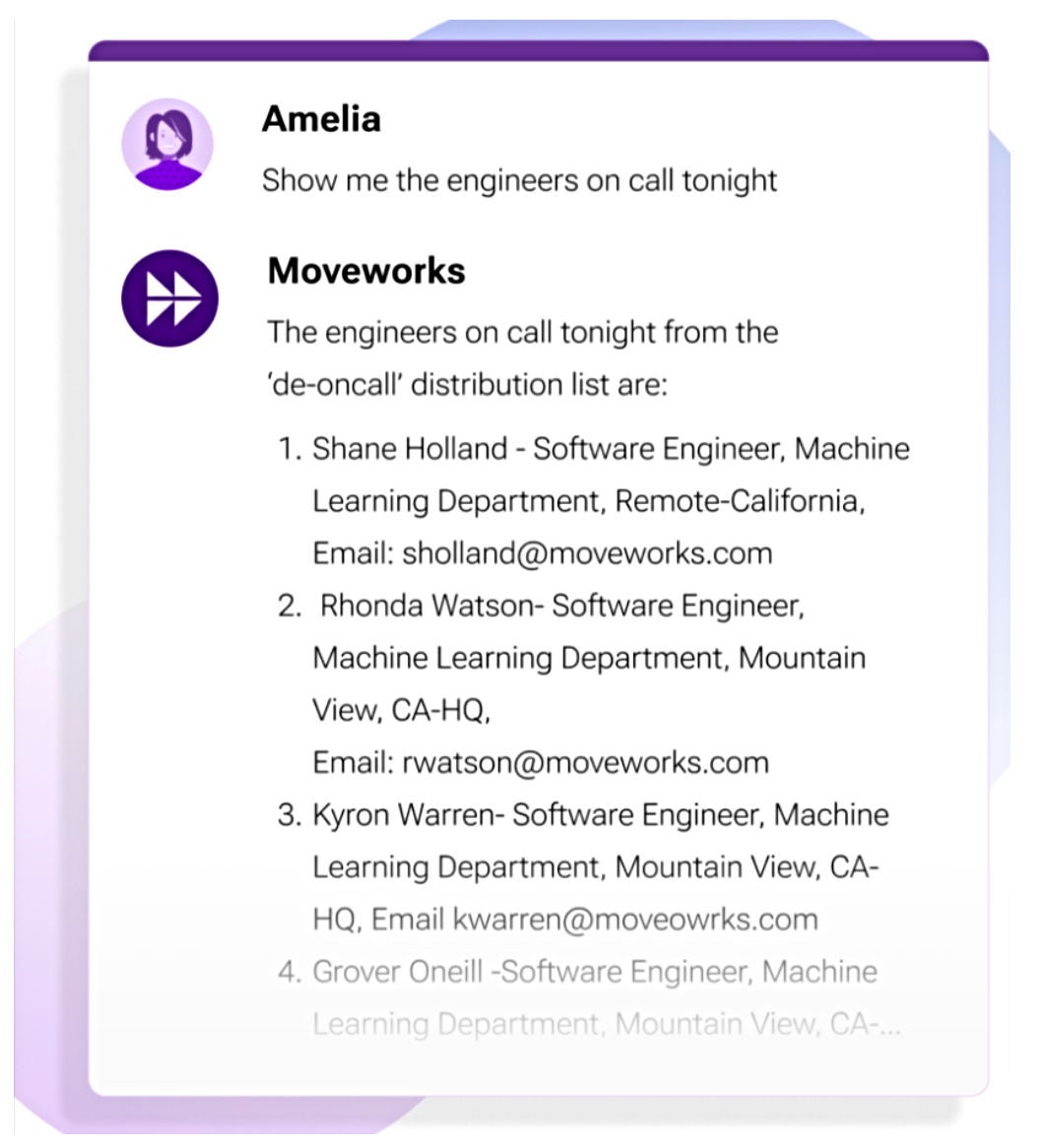
Agentic AI for engineering goes far beyond what traditional—or even generative—AI can do alone. Traditional AI can help identify problems and handle simple tasks, but it struggles to navigate more complex engineering workflows like publishing and deployment.
In contrast, agentic AI can help provision system resources if unexpected usage is detected, automatically find potential software bugs if a problem gets logged, and notify engineering teams of potential outages before they occur. In turn, engineering teams can become more proactive, agile, and innovative, driving business success.
6. Customer service
Large organizations get numerous customer service requests each day—many of them requiring employees to perform repetitive tasks. You may be able to use traditional AI to help with some of these tasks, like answering simple questions and drafting sample responses, but your team must still determine the best course of action and manually carry it out.
AI agents have access to much more data and can use that data to perform complex tasks. For example, a customer service agent may need to get order information from a customer. Instead of manually logging into order systems, the representative can ask an AI chatbot to retrieve the information, look for problems, and automatically rectify them without human input.
Quick tips on adopting and implementing agentic AI
Choosing an agentic AI solution to leverage in your business won’t be an easy decision, nor should it be. The solution you choose will integrate into your company’s broader digital transformation, and it’s a strategic investment that will help shape your company’s future.
Start by aligning your AI strategy with your company’s overarching goals—whether it’s improving productivity, finding ways to cut costs, or scaling services across departments. Consider where AI can deliver the most value and ensure its use cases support your organization’s long-term roadmap.
There are many considerations to think about when making your choice:
- Does your AI agent align with your company’s governance framework? If not, can you customize your agent or make changes internally to bring them into alignment?
- Are you able to utilize human oversight with your AI agent to guide its goals and decisions?
- Can you test your AI agents to catch potential issues and find edge cases?
- Are you able to implement controls and restraints to ensure the autonomy of your agents is restrained to specific tasks and domains?
This decision shouldn’t be one that your IT department makes alone. Bring all your stakeholders together—including IT, compliance, HR, finance, and other departments. Your teams should work collaboratively to guide the adoption of AI agents and continuously refine your requirements for performance optimization.
With transparent reasoning frameworks and regular checks on performance, your enterprise can build trust in your agentic AI systems, ensuring your solutions are powerful and aligned with your organization’s goals.
Top 3 challenges & risks to consider before deploying an AI agent
Enterprises should deploy AI agents only after considering the challenges they present. AI agents are powerful tools, but deployed the wrong way, they can cause problems that may be difficult to overcome. With that in mind, use these strategies to minimize risks when adopting an enterprise AI solution:
Prioritize security: Agentic AI systems often handle sensitive data and execute actions that have far-reaching consequences. Implement robust security measures by deploying authentication measures, encryption, secure transfers, and strict access controls. Keep monitoring system logs to identify potential security issues and unauthorized activity in real time.
Provide testing and human supervision: It’s important to properly test AI agents before deploying them at scale. AI agents are statistical models, so they take action based on what they calculate is the proper choice—but that won’t always be the right choice in some edge cases. Incorporate human review processes so trusted teams can intervene and guide the AI’s evolution.
Look at reasoning: One of the biggest problems with generative AI models is that they are a black box—it’s hard to know how generative AI models arrive at conclusions, which makes them harder to trust. Transparent reasoning can help stakeholders understand why AI agents take the actions they do. As much as possible, build systems that require the AI agents to lay out their reasoning behind actions, log those decisions, and provide them to trusted team members to review the AI’s reasoning process.
Why enterprises can’t afford to wait to invest in agentic AI
Delaying agentic AI deployment means missing out on the transformative benefits that AI brings to the table. It isn’t a small change, either—reports show that AI may increase corporate profits by $1.4 trillion per year.
The biggest challenge many organizations will face is implementation. Integrating a comprehensive AI solution into your current workflows takes time and effort—but that’s not a reason to avoid starting now, especially when 82% of companies plan to implement AI agents within the next three years.
With its advanced reasoning and analytical abilities, agentic AI has the potential to completely reshape how enterprises run when implemented properly. Your teams will work more efficiently and have more time to focus on critical tasks, leading to increasingly streamlined operations.
Start building your future with agentic AI
AI is evolving at breakneck speed, and agentic AI is one of the most promising technologies for enterprises. As AI capabilities continue to evolve, expect its capabilities to grow—as well as the benefits it can deliver to enterprises.
Agentic AI enables your business to dynamically respond to changing market conditions, refine processes and operations, and leverage data-driven insights—which can translate into a clear competitive advantage.
Moveworks stands at the forefront of the agentic AI space with our comprehensive enterprise AI Copilot solution, which is powered by an Agentic Automation Engine that makes potent agentic AI abilities accessible across your entire organization. Moveworks also enables you to quickly build and deploy AI agents at scale with our robust developer platform, so you can easily customize automations for your unique business needs.
Featuring robust security and integrations, along with a user-friendly and intuitive interface, Moveworks delivers the complete solution your enterprise needs to leverage the full benefits of agentic AI. Our agentic architecture turns employee input into tangible business outcomes.
Ready to experience it yourself? Try the Moveworks Playground or get a demo to see Moveworks in action.
The rise of new AI-powered tools is changing the way organizations operate. And for enterprises, agentic AI can transform your business at a fundamental level.
JPMorgan Chase can attest to the power of agentic AI—it’s already saving the organization 360,000 hours of manual review a year. As can AT&T, which reduced operating expenses by 15%. Meanwhile, Amazon and Netflix used agentic AI to increase customer retention by 20% and 10%, respectively.
If your enterprise isn’t preparing to join these AI leaders, you risk falling behind the competition.
What is agentic AI?
Agentic AI refers to artificial intelligence systems that have goal-directed behavior and can take autonomous actions to achieve those goals. In other words, agentic AI solutions are designed to take initiative and set and pursue goals, while continuously learning and adapting based on new information.
Table of contents