In the race to harness artificial intelligence (AI), a burning question looms: Do AI investments really pay off?
With the advent of AI, the world is witnessing a massive technological shift. Last year, a tipping point was reached, with over half of organizations experimenting with generative AI (genAI) in their workflows. Yet, studies reveal that most AI projects still struggle to achieve profitability, with returns falling short of the typical cost of capital.
Nonetheless, the power of high-quality data and mature AI capabilities are evident, with best-in-class companies reporting impressive return on investment (ROI) from their AI initiatives. Businesses are eagerly pouring in resources, venture capitalists are happily placing their bets, and the employees are rapidly putting AI tools to practical use. Driven by the promise of unprecedented efficiency, enhanced customer experience, and a definite competitive edge, the allure of AI is undeniable.
However, as investments soar and companies hop on to the AI bandwagon, it becomes more important than ever to determine if these investments are really justified. Is the frenzy worth the hype?
To be sure — generative AI is a paradigm-shifting technology that has the potential to transform entire industries and revolutionize the way we approach problem solving but it’s only as valuable as its real-world application.
In this blog, we will cover:
- The definition of ROI of AI
- ROI: Hard versus soft returns
- Challenges in measuring the ROI of AI
- Strategies to optimize and maximize AI investments
- The promise of an AI copilot
- To build or not to build?
The definition of ROI of AI
In simple terms, ROI is essentially a financial ratio of gain or loss of an investment relative to its cost. It is measured as net return divided by the original capital cost of the investment. The higher the ratio, the greater the benefit.
The ROI of AI or the return on investment of artificial intelligence, however, is defined as the value gained, the experience delivered or the profit realized as a result of implementing AI technologies and solutions.
Unlike the return on traditional investments where gains are compared against costs for immediate financial returns, the return on AI investments may not necessarily have a financial impact in the short term. Rather, benefits arising from self-service, automation of tasks, and predictive analytics have a far reaching impact on employee productivity and robust decision making. These tend to accumulate over time and contribute to long-term business success.
According to Gartner, “Generative AI promises unprecedented productivity improvements and business transformation opportunities, but calculating the value of new investments in GenAI requires you to build a business case by simulating potential cost and value realization across a range of GenAI activities.” These typically include quick wins, differentiating use cases, and transformational initiatives.
ROI: Hard versus soft returns
Management consulting firm, McKinsey & Company, asserts that: “Generative AI is poised to unleash the next wave of productivity,” emphasizing the economic potential of its overarching impact on productivity. Their latest research “estimates that generative AI could add the equivalent of $2.6 trillion to $4.4 trillion annually. This would increase the impact of all artificial intelligence by 15 to 40 percent.”
The ROI of AI investment results in both tangible and intangible benefits termed as hard versus soft returns. The hard returns are quantifiable in monetary value terms and directly attributable to the investment, while soft returns are indirect contributors to business value and growth. We will examine both in the following section.
What are hard returns of AI investments?
At a fundamental level, the hard returns of artificial intelligence have direct linkages to profitability. These include metrics such as increase in revenue, reduction in cost, savings in time, and mitigation of risks.
By analyzing large data sets, automating repetitive tasks, and enabling self service, AI investments can help enterprises unlock new streams of revenue, optimize existing investments, reduce operational costs, identify potential risks, and make more informed business decisions.
In the healthcare vertical, a practical example of hard returns could be freeing up the time of data scientists to work on molecules that could go on to save human lives. In the e-commerce vertical, the deployment of conversational AI led to a 74% reduction in ticket volume at Mercari, which in turn allowed the company to reduce technical debt and scale its operations.
What are soft returns of AI investments?
Soft returns of AI investments look at a broader set of benefits and gains that accrue over time. They may not have an impact on profitability or revenue of the company in the near term but they are vital and cannot be discounted. These metrics include an uptick in employee engagement, end-user productivity, customer experience, and enterprise innovation.
By taking the friction out of work and streamlining workflows and processes, the employee retention metric trends upward — the workforce becomes happier, more engaged, and productive. This leads to happier customers, less churn, more innovation, and increase in their lifetime value.
For example, Pinterest was able to transform its employee experience and achieve a 99% reduction in issues rate for its top communication campaign by leveraging an AI bot. Stuck in a failed communication loop at one point, the social media giant is now able to target specific employees, send them dynamic messages via Slack, and help them take action in real time.
Challenges in measuring the ROI of AI
Difficulties stemming from the lack of measurable key performance indicators (KPIs), holistic vision and strategy, data quality, and cost of implementation can make the measurement of ROI particularly challenging. Research indicates that 82 to 93 percent of AI projects fail which means you need to tread with caution. As digital transformation initiatives continue to fail, you want to consider the opportunity cost and be sure your investments are going to pay off before pouring in huge sums of money.
The three common pitfalls that have historically plagued AI investments and digital transformation initiatives are:
✔ Lack of holistic strategy and goals
✔ Lack of vision alignment across business and technology functions
✔ Lack of monitoring of success, outcomes, and measurable KPIs
These challenges can result from a wide array of factors such as complexity of projects, upfront investments, unrealistic expectations, discounting tangible benefits, the continuously evolving business environment, conflicting priorities, the time taken to realize some of these returns, and the lack of methodologies to measure and evaluate impact. It’s also worthwhile to keep in mind that output is only as good as input and poor data quality can adversely affect the efficacy of ROI calculations.
In addition to this, organizations also rely on survey-based approaches. While common, this approach leads to a superficial understanding of effectiveness. It often relies on questions like: "Did you get value from the program?" While such surveys can gauge satisfaction, they lack the detail to pinpoint what the program achieved, or failed to achieve, or how it performed against the overarching goals. This can lack the depth of the outcome-based approach which we will discuss later.
According to PwC, the three big mistakes that organizations should guard against as they make investments in AI are:
✔ Discounting the uncertainty of benefits
✔ Computing ROI based on a point in time
✔ Treating each AI project individually
It, therefore, becomes imperative that the enterprise goes beyond simple ROI calculations, takes into account the uncertainties, estimates error rates, measures AI’s performance on a continual basis, budgets for maintenance, and considers their company’s entire portfolio of AI projects, at any given point.
Addressing these challenges requires careful planning, robust measurement frameworks, and ongoing evaluation to ensure that ROI calculations accurately reflect the value generated by AI implementation.
Strategies to optimize and maximize AI investments
A Microsoft-sponsored market study indicates a 3.5X return on AI investments with 5% of the organizations worldwide realizing as much as 8X. For this study, IDC was commissioned to survey more than 2,000 business leaders responsible for bringing AI transformation within their organizations. It provides unique insights into expected returns and the business value of AI.
Next, let’s explore tips and strategies for maximizing and optimizing the value of AI investments.
An IBM study outlines six core AI capabilities needed to maximize AI investments. These include: vision and strategy, data and technology, AI operating model, talent and skills, AI engineering and operations, and culture and adoption.
At Moveworks, we’ve identified the three key pillars for successful AI transformation as business outcomes, technology, and operating model — and it’s at the intersection of the three that the magic happens. There needs to be a clear linkage of technical use cases to outcomes, value, and measurable KPIs along with platform capabilities tightly aligned to capacity needs. From an operational perspective, delivery processes, operations, enablement, analytics, and change management need to be clearly defined.
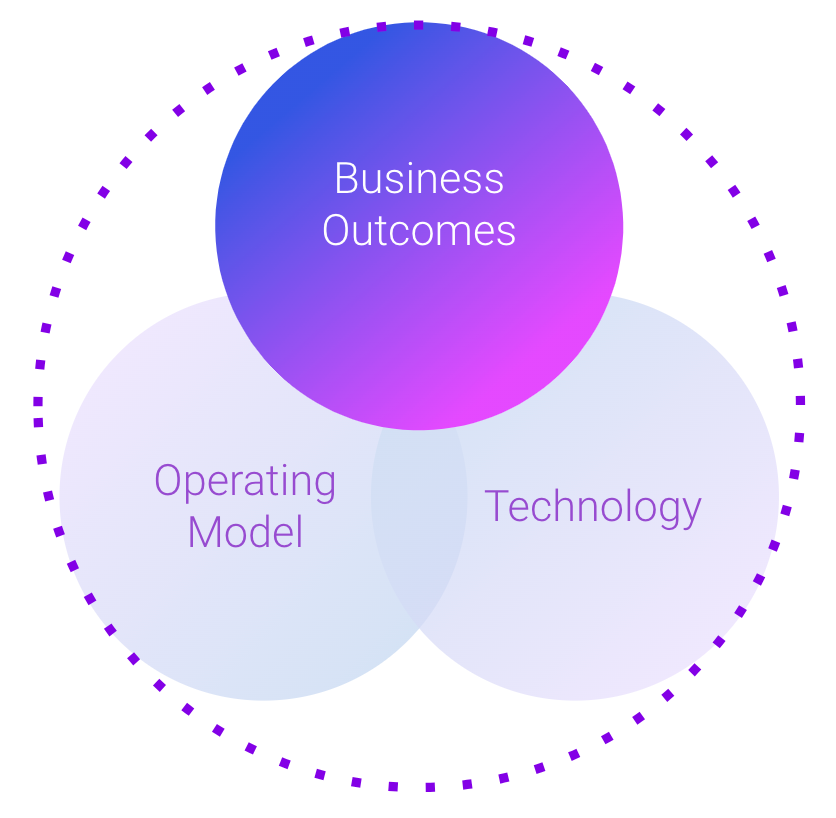
Moving towards an outcomes based approach
Aligning AI investments to business outcomes lays the foundation for optimizing and maximizing investments in artificial intelligence. The outcomes based approach involves identifying the business challenges and objectives, defining specific use cases, and establishing the success criteria — all in collaboration between technology and business teams. It is also worth assessing the level of effort, technology, people, processes, and data capabilities required at the very onset.
The four key elements of the Moveworks ‘Business Outcomes Framework’ are outlined below:
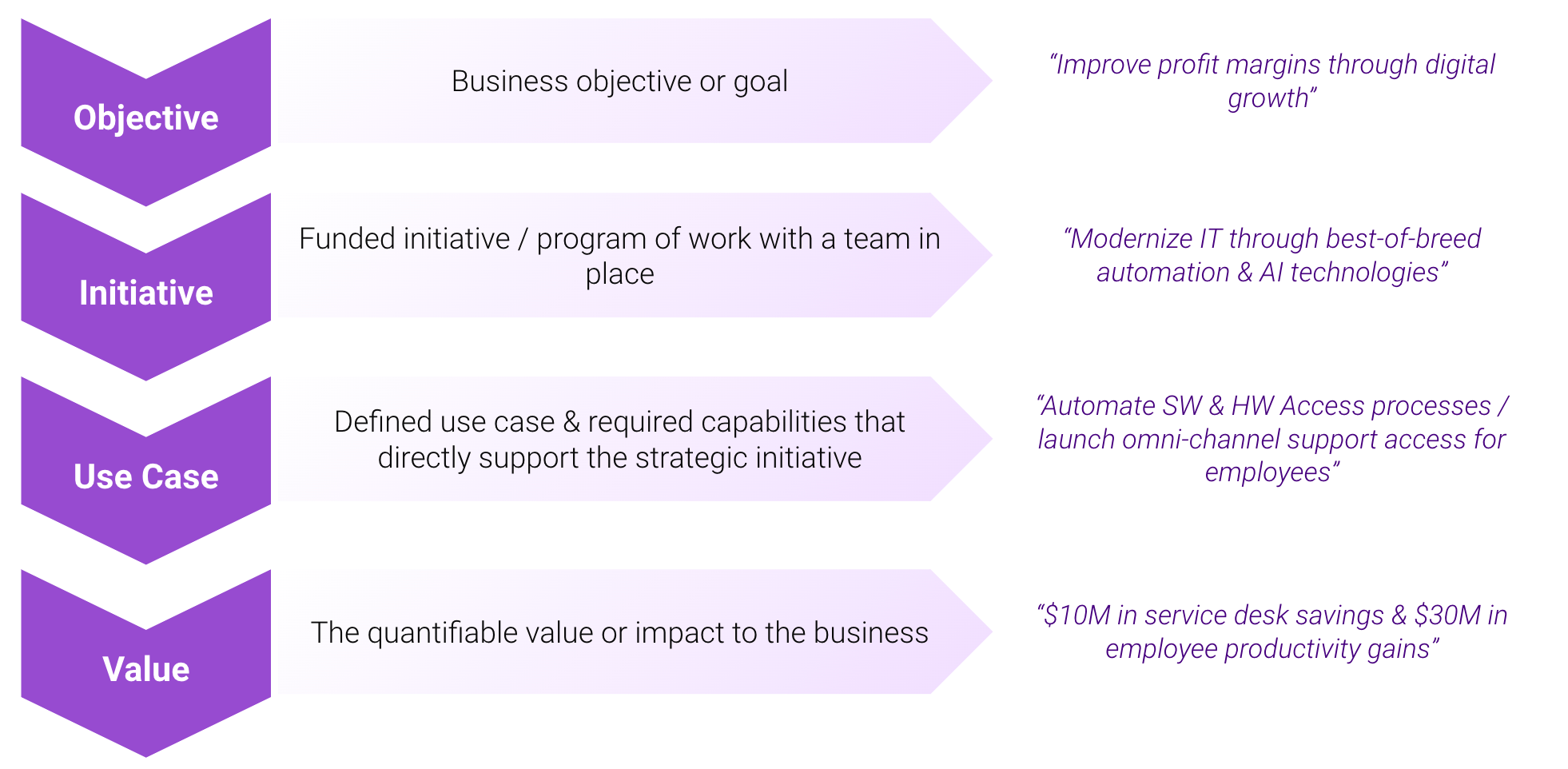
This should enable organizations to identify important AI use cases while determining their value or ROI to the business. Furthermore, the Moveworks’ AI value framework is grounded in three key business outcomes namely:
✔ Improving operational efficiency
✔ Enhancing experiences
✔ Accelerating business transformation
At a time when productivity has become synonymous with profitability, it becomes more critical than ever for organizations to effectively and efficiently utilize its resources be it time, people, or capital to achieve its goals while delivering delightful, optimal experiences across employees, partners, and customers. The market dynamic might even call for business transformation that involves fundamentally changing how your organization operates to improve its performance and competitiveness.
The promise of an AI copilot
As organizations move towards an outcomes based approach, it becomes vital to identify the business objectives, define key use cases and capabilities, and establish a value framework. The framework should include specific KPIs from the key pillars to inform initial and ongoing AI investments. At Moveworks, we have developed a four-tiered framework to help business leaders better understand the technology and investments necessary to integrate LLMs into production environments.
Before we delve into the four tiers, let’s do a quick refresh on the AI copilot. An AI copilot is a powerful tool or a conversational interface that leverages large language models (LLMs) within an enterprise environment. It can automate tasks and retrieve information across multiple domains, applications, and business systems. Furthermore, it possesses the capability to understand, analyze, and process vast amounts of data.
AI copilots are built on two key components: artificial intelligence and integrations across systems. The AI algorithms enable copilots to predict user needs, define context, and provide relevant answers. Through integrations, AI copilots can interact with a vast range of systems, creating a unified, interconnected platform for seamless communication and task management.
The Moveworks’ four-tiered framework, at a glance:
✔ A tier-one copilot involves a simple LLM integration that leverages basic API calls.
✔ A tier-two copilot calls for customized LLM integration, grounded in an organization’s domain specific data.
✔ A tier-three copilot involves chaining multiple LLMs together, creating sophisticated pipelines optimized for multi-step use cases.
✔ A tier-four copilot is a sophisticated LLM system specifically designed for enterprise-wide deployment with advanced features like a reasoning engine, analytics, connectors, security, and privacy.
The diagram below maps the use cases pertaining to different tiered copilots with the investment requirement on the x-axis and the scope on the y-axis:
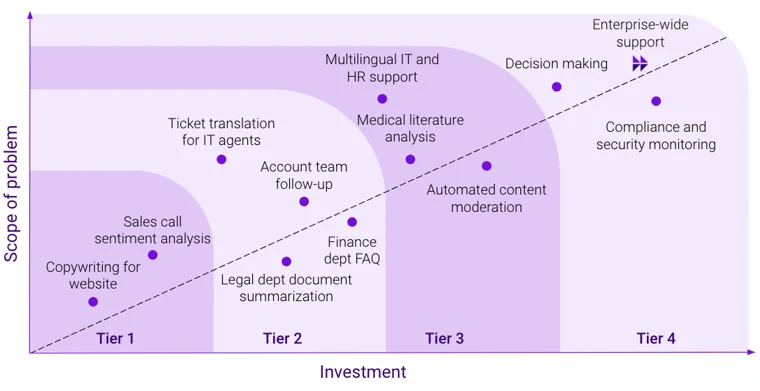
A tier-four or an enterprise grade AI copilot has the potential to make the service desk more efficient, automate tasks, reduce manual processes, improve employee productivity, provide onboarding support, minimize churn, and enhance the security posture of an organization.
To build or not to build?
According to the Gartner research stated above, “By [the year] 2028, more than 50% of enterprises that have built AI models from scratch will abandon their efforts due to costs, complexity, and technical debt in their deployments.”
This brings us to the quintessential question of build versus buy. Answering this requires a careful evaluation of your goals and outcomes. Are you looking for a basic model or an enterprise grade edition? In that light, you’d want to consider the pros and cons of either building or buying an AI copilot in terms of cost, complexity, expertise, resources, time, infrastructure, control, processes, and investment.
To conclude, businesses need to approach AI investments strategically, considering both hard and soft returns. In order to maximize their AI returns, factors such as strategic alignment, articulation of business objectives and outcomes, establishing meaningful use cases and KPIs, and building an operating model around value and impact become key considerations. Get ready to embark on your AI journey with our build versus buy guide on your side.
Looking for an in-depth resource to inform your build versus buy strategy? Download the Moveworks guide now.
Table of contents