Every day hundreds of millions of employees across the world get stuck when they can’t get the right information and insights they need to complete their tasks. That’s because data exists in many different formats, and is siloed across hundreds, if not thousands of business applications, each with a different set of permissions and access controls. This enduring problem of finding information has led to wasted hours and lower employee productivity across the board.
The arrival of Retrieval Augmented Generation (RAG)
The first large-scale impact of generative AI came with ChatGPT, which instantly transformed the search experience using large language models (LLMs) and Retrieval Augmented Generation (RAG). RAG enabled LLMs to be linked to information sources so users could interact with source systems through a chat interface. Users could query the underlying sources to get answers grounded in the retrieved data sources.
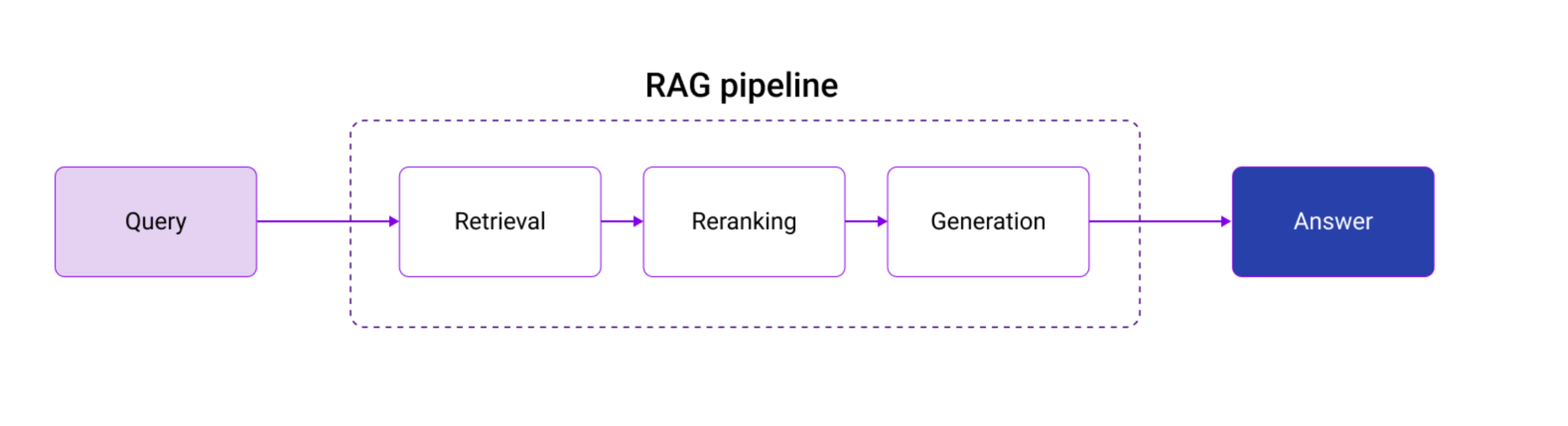
Enterprises quickly realized the potential of RAG – it was easy to prototype internally and offered a dramatic improvement in quality and coherence over keyword-based search. Search no longer was just a wall of weakly relevant blue links with scattered blocks of information - and instead, employees saw summaries grounded in data.
But as organizations tried to scale and operationalize these RAG solutions, they discovered core problems with this architecture which failed when the scale of data and overlap of information increased. So, even though RAG was a great first step, the fundamental friction around finding diverse, relevant information across many systems continued to exist.
RAG alone is not enough for Enterprise Search
Enterprise-wide adoption of RAG has been limited due to two fundamental shortcomings: the challenge of accuracy and the challenge of hallucinations. These two factors have a direct role in search quality, which is the primary measure of success for any search solution.
Challenge of Accuracy
Results are meaningless if they’re not accurate. What often creates impediments here is that overlapping information exists across diverse sources of data. Imagine a salesperson looking for the revenue on an opportunity. Employees know that a CRM is generally the most trustworthy source of revenue data. But how does a RAG system know what is a trustworthy source given a Teams or Slack chat, email from the VP of Sales, or a figure from the CRM?
As a result, it pulls updates from an email instead and potentially misleads an employee. In fact, this scenario was cited by Microsoft’s head of AI products as a real challenge for RAG systems.
Challenge of Hallucinations
Hallucinations are an inherent property of LLMs. The superpower of LLMs is natural and persuasive language generation due to reinforcement learning via human feedback. It’s what makes them so attractive, but is also their Achilles heel if not addressed properly.
When summarizing content, LLMs can make up information or distort facts to fill in information gaps in order to appear coherent and persuasive. This militates against the core value proposition of accuracy of information - especially in the context of enterprise search.
A quick search will show many examples of failed RAG systems:
- At Cargill, AI failed to correctly answer a question about who is on the company’s executive team
- At Eli Lilly, AI gave incorrect answers to questions about expense policies
This shortcoming can be especially harmful or destructive as RAG systems can mislead users with AI-generated summaries. Grounding, which is the process of generating text by providing content to summarize, is necessary, but nowhere sufficient to prevent hallucinations. RAG systems don’t have architectural capabilities that can impose grounding in their results.
The daunting scale of retrieval of enterprise data
We’ve seen search gain traction among consumers, but the same cannot be said for organizations. Beyond the architectural challenges of RAG, there’s also additional layers of requirements needed for a search solution to be enterprise-ready.
- Challenge of scale (volume and variety): Companies often have terabytes to petabytes of data within billions of files, documents, and business objects. Teams get stuck on the data infrastructure required to handle this volume and variety of data.
- Challenge of permissions: Enterprise applications and data sources have different permissions associated with underlying content, which is integral for business operations. Most RAG-based search systems falter due to a lack of respect for complex permissions and access controls.
- Challenge of integrations: Enterprises can have anywhere from dozens to thousands of applications and data sources. When RAG systems don’t have these deep integrations or easy-to-use tools to configure new connectors, it’s difficult to provide real enterprise-wide value.
- Challenge of business context: Basic RAG systems don’t have any awareness of unique business context. Sometimes, internal jargon and acronyms get mixed up as the keyword or even spell-corrected by LLMs. For a user, this degrades the quality of the results, especially when they are looking for business-specific information and insights.
- Challenge of governance and analytics: After the rollout of an enterprise search solution, businesses need visibility into how it’s used. Without it, stakeholders won’t know what they can’t measure – relying on a best guess effort that the solution is performing well and that results are generally useful and accurate.
A new way forward: Agentic RAG delivers insights that RAG could not
It’s clear that enterprise search needs to take full advantage of the groundbreaking advances in LLMs and language technologies, while also being enterprise-ready. We need to rethink enterprise search as less of a shallow, simplistic way to retrieve and summarize information, and more as a dynamic, intelligent, and goal-oriented system which checks its results for accuracy before providing an answer to the user. Employees aren’t simply searching for information, they are researching for answers and insights.
That’s where Agentic AI comes in.
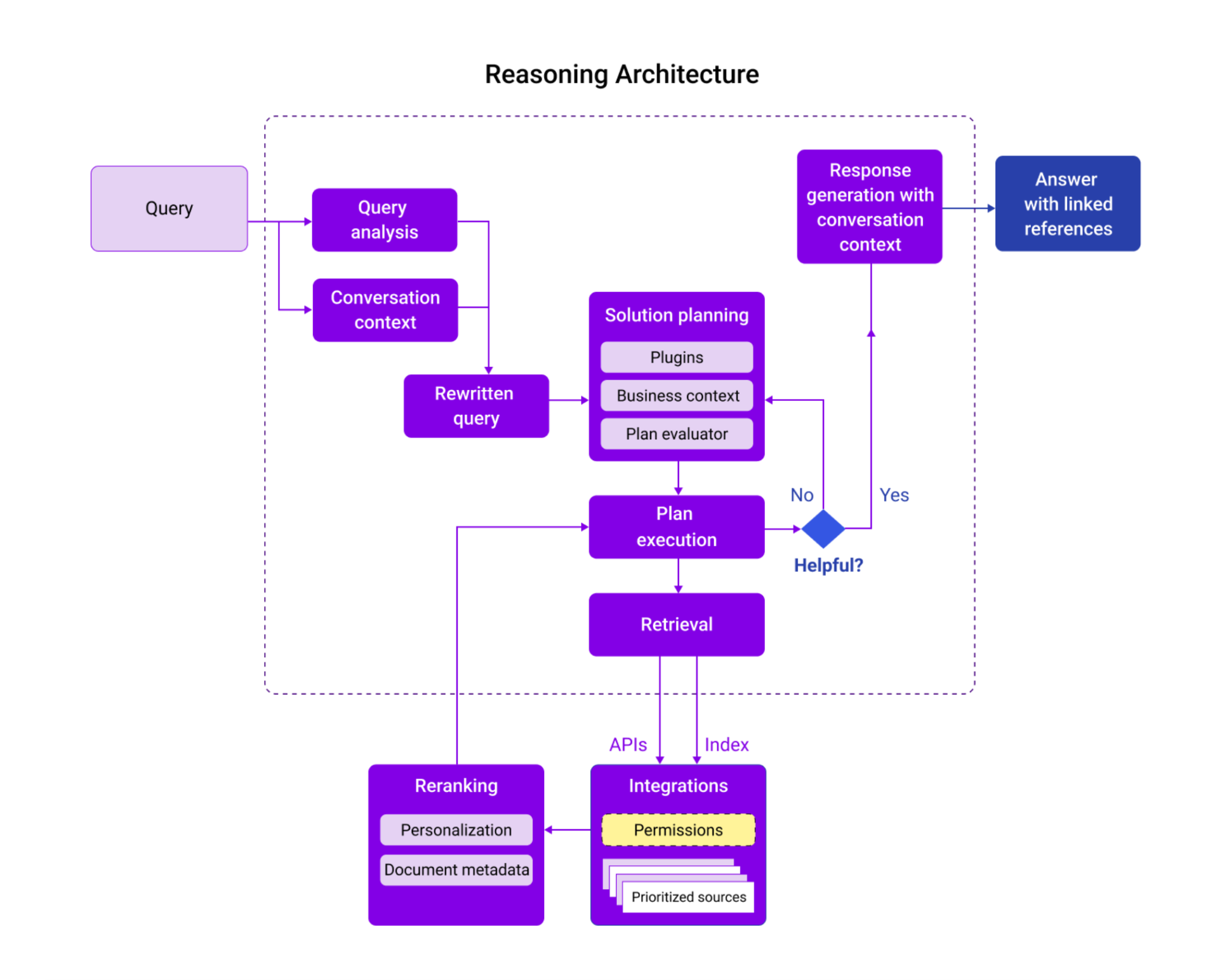
Agentic RAG combines Agentic AI with RAG. It brings reasoning to search to deliver enhanced quality and accuracy. For every query, an agentic RAG system performs a few key steps: understanding the user's objective, query enrichment and planning, intelligent retrieval and ranking, and direct & reflected summaries.
It transforms enterprise search with a goal-driven, autonomous decision-making approach to knowledge retrieval. The added layers of intelligence are what differentiates Agentic RAG from basic RAG so that:
- It’s personalized and can predict what the user wants based on analyzing their prompt as well as attributes like their role, identity, location, etc.
- It’s directed and can prioritize systems and content based on the user’s prompt and type of query.
- It uses context to understand the relative importance and reliability of data sources, such as content recency, system, activity signals like most viewed or highly rated, and more.
Delivering on this new way forward with agentic RAG unlocks significant upside for employees. They get answers that are more accurate, trustworthy, and personalized at scale. These are critical enhancements necessary to drive meaningful adoption of enterprise search.
Introducing Moveworks Enterprise Search
Moveworks Enterprise Search leverages agentic AI to deliver the trustworthy and accurate answers that employees are actually looking for.
Moveworks is bringing a new approach to enterprise search by enhancing RAG with reasoning. It leverages a powerful Reasoning Engine that’s able to understand employee goals, develop intelligent plans, and search across various business systems to return top-quality search results. Here’s how:
- Agentic RAG delivers better accuracy and reliability of answers to help drive the ubiquitous adoption of a search solution.
- Citations presence that makes every effort to fact-check for accuracy and link to citations where possible to help to solve for the distortion of information through hallucination.
- Over 100+ connectors that integrate Moveworks Enterprise Search to the most useful information repositories, as well as a way to add connectors to custom content systems.
- Granular permissions and access controls help ensure the right employees have access to the right information (and nothing more).
- Data volume and variety. Moveworks Enterprise Search scales to support the large data volumes and variety of business objects commonplace in the enterprise.
- Robust analytics to enable stakeholders to stay in the loop on adoption as well as usage, and make data-driven decisions on how to continuously improve the overall state of service for employees.
Together, these features formulate a solution that’s able to be enterprise-ready from day one. Moveworks Enterprise Search will finally help deliver the reliability, scalability, and security demands of organizations today. It’s time to take search to the next level. Break free from obsolete, basic RAG and get ready to jump aboard enterprise search powered by agentic RAG with Moveworks Enterprise Search.
What to learn more about the power of Enterprise Search? Learn more about our solution at our virtual live event on November 12 at 10AM PT.
Table of contents